The Full Guide to Quant Funds: Understanding the Basics and Beyond
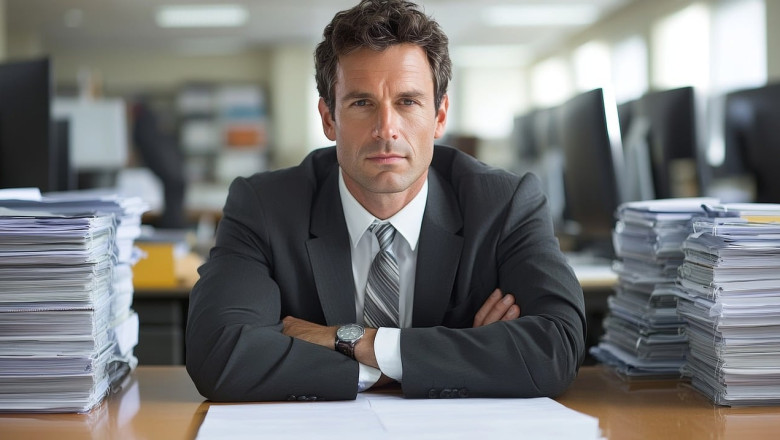
The Full Guide to Quant Funds: Understanding the Basics and Beyond
Introduction to Quantitative Funds
Definition and Overview
Quantitative funds, often referred to as “quant funds,” are investment funds that utilize mathematical models and algorithms to inform their trading decisions. These funds rely on quantitative analysis, which involves the use of statistical and mathematical models to evaluate financial data and identify trading opportunities. The primary goal of quant funds is to leverage data-driven insights to achieve superior returns while managing risk effectively.
Quant funds can operate across various asset classes, including equities, fixed income, commodities, and currencies. They employ a range of strategies, such as statistical arbitrage, trend following, and market-neutral strategies, to capitalize on market inefficiencies. The use of technology and advanced computing power is a hallmark of quant funds, enabling them to process vast amounts of data and execute trades at high speeds.
The appeal of quant funds lies in their systematic approach, which reduces the influence of human emotions and biases in investment decisions. By relying on data and models, quant funds aim to achieve consistent performance and adapt to changing market conditions.
Historical Context and Evolution
The origins of quantitative investing can be traced back to the mid-20th century, with the development of modern portfolio theory and the efficient market hypothesis. These foundational theories laid the groundwork for the use of quantitative methods in finance. The advent of computers in the 1960s and 1970s further accelerated the growth of quantitative analysis, as it allowed for the processing of large datasets and the implementation of complex models.
The 1980s and 1990s saw the rise of prominent quant funds, such as Renaissance Technologies and D.E. Shaw, which demonstrated the potential of quantitative strategies to deliver exceptional returns. These firms attracted top talent from fields such as mathematics, physics, and computer science, further advancing the sophistication of quantitative models.
In the 2000s, the proliferation of data and advancements in technology, including machine learning and artificial intelligence, transformed the landscape of quantitative investing. Quant funds began to incorporate alternative data sources, such as social media sentiment and satellite imagery, to enhance their models and gain a competitive edge.
Despite their success, quant funds have faced challenges, including periods of underperformance and market disruptions. The 2007-2008 financial crisis, for example, highlighted the limitations of some quantitative models and underscored the importance of risk management.
Today, quant funds continue to evolve, leveraging cutting-edge technology and data science techniques to refine their strategies and adapt to an ever-changing market environment. As the field of quantitative investing grows, it remains a dynamic and integral part of the financial industry.
How Quant Funds Work
The Role of Algorithms and Data
Quantitative funds, commonly known as quant funds, rely heavily on sophisticated algorithms and vast amounts of data to make investment decisions. At the core of a quant fund’s operation is the use of mathematical models and statistical techniques to analyze financial data and identify trading opportunities. These algorithms are designed to process large datasets, which can include historical price data, financial statements, economic indicators, and even alternative data sources like social media sentiment or satellite imagery.
The algorithms employed by quant funds are typically developed by teams of quantitative analysts, data scientists, and financial engineers. These professionals use advanced programming languages and software tools to create models that can identify patterns, correlations, and anomalies in the data. The models are then backtested using historical data to evaluate their performance and refine their predictive capabilities.
Data is the lifeblood of quant funds, and the quality, volume, and timeliness of data can significantly impact the fund’s performance. Quant funds often invest in acquiring high-quality data feeds and employ data cleaning and preprocessing techniques to ensure the data is accurate and usable. The ability to process and analyze data in real-time is also crucial, as it allows quant funds to react quickly to market changes and execute trades at optimal times.
Key Strategies Employed
Quant funds employ a variety of strategies to achieve their investment objectives, each leveraging the power of algorithms and data in different ways. Some of the key strategies include:
Statistical Arbitrage
Statistical arbitrage involves identifying and exploiting pricing inefficiencies between related financial instruments. Quant funds use statistical models to detect temporary mispricings and execute trades to profit from the expected convergence of prices. This strategy often involves high-frequency trading and requires sophisticated algorithms to identify opportunities and execute trades rapidly.
Trend Following
Trend following strategies aim to capitalize on the momentum of asset prices. Quant funds using this approach analyze historical price data to identify trends and make trades that align with the direction of the trend. These strategies can be applied across various asset classes, including equities, commodities, and currencies, and often involve the use of technical indicators and moving averages.
Mean Reversion
Mean reversion strategies are based on the idea that asset prices tend to revert to their historical averages over time. Quant funds employing this strategy look for assets that have deviated significantly from their average price and place trades anticipating a return to the mean. This approach requires careful analysis of historical price data and the use of statistical models to determine the likelihood of reversion.
Machine Learning and AI
Some quant funds are at the forefront of incorporating machine learning and artificial intelligence into their investment processes. These funds use advanced machine learning algorithms to analyze complex datasets and uncover patterns that traditional models might miss. Machine learning can enhance the predictive power of quant models and enable funds to adapt to changing market conditions more effectively.
Factor Investing
Factor investing involves targeting specific drivers of returns, known as factors, such as value, momentum, size, or quality. Quant funds use data analysis to identify and invest in securities that exhibit desirable factor characteristics. This strategy requires a deep understanding of the factors that influence asset prices and the ability to construct portfolios that optimize exposure to these factors.
Each of these strategies requires a robust infrastructure for data processing, model development, and trade execution. Quant funds continuously refine their models and strategies to adapt to evolving market conditions and maintain a competitive edge.
Types of Quantitative Strategies
Statistical Arbitrage
Statistical arbitrage, often referred to as “stat arb,” is a quantitative strategy that seeks to exploit pricing inefficiencies between related financial instruments. This strategy relies heavily on mathematical models and statistical techniques to identify and capitalize on these inefficiencies. The core idea is to take advantage of mean-reverting behaviors in asset prices, where the price of an asset is expected to return to its historical average over time.
Statistical arbitrage strategies typically involve pairs trading, where two correlated securities are traded against each other. For example, if two stocks historically move together but temporarily diverge, a statistical arbitrageur might short the overperforming stock and go long on the underperforming one, betting that the prices will converge again. This strategy requires sophisticated algorithms to analyze large datasets and identify potential opportunities quickly.
Risk management is crucial in statistical arbitrage, as the models are based on historical data and assumptions that may not always hold true. The strategy often involves high-frequency trading and requires significant computational power and data analysis capabilities.
Trend Following
Trend following is a quantitative strategy that aims to capitalize on the momentum of asset prices. The basic premise is that assets that have been rising in price will continue to rise, and those that have been falling will continue to fall. This strategy is grounded in the belief that markets exhibit trends due to various factors, such as investor behavior, economic conditions, and market sentiment.
Trend following strategies use technical indicators and algorithms to identify and follow these trends. Common tools include moving averages, momentum indicators, and breakout signals. The strategy is often applied across various asset classes, including equities, commodities, currencies, and futures.
One of the key advantages of trend following is its simplicity and adaptability to different market conditions. However, it can also be prone to false signals and whipsaws, where prices reverse quickly, leading to potential losses. Effective risk management and diversification are essential components of a successful trend following strategy.
Market Neutral
Market neutral strategies aim to generate returns regardless of overall market movements by balancing long and short positions. The goal is to eliminate or minimize market risk, focusing instead on the relative performance of individual securities or sectors. This approach seeks to profit from the spread between the long and short positions, rather than the direction of the market.
Market neutral strategies often involve pairs trading, similar to statistical arbitrage, but with a focus on maintaining a balanced exposure to the market. For example, a market neutral fund might go long on undervalued stocks while shorting overvalued ones within the same sector, aiming to profit from the relative price movements.
These strategies require sophisticated risk management techniques to ensure that the portfolio remains market neutral. This involves continuously monitoring and adjusting the portfolio to maintain the desired balance. Market neutral strategies can be particularly appealing in volatile or uncertain market environments, as they are designed to reduce exposure to broad market risks.
Advantages of Quant Funds
Data-Driven Decision Making
Quantitative funds, or quant funds, leverage sophisticated mathematical models and algorithms to make investment decisions. This data-driven approach offers several advantages:
Objective Analysis
Quant funds rely on objective data analysis, minimizing the influence of human emotions and biases that can often cloud judgment in traditional investment strategies. By using historical data and statistical models, quant funds can identify patterns and trends that may not be immediately apparent to human analysts.
Speed and Efficiency
The use of algorithms allows quant funds to process vast amounts of data quickly and efficiently. This capability enables them to react to market changes in real-time, executing trades at speeds that are impossible for human traders. This speed can be crucial in capturing short-term market inefficiencies and opportunities.
Consistency
Quantitative models are designed to follow a set of predefined rules, ensuring a consistent approach to investment decisions. This consistency can lead to more predictable performance over time, as the models are not swayed by market noise or short-term fluctuations.
Diversification and Risk Management
Quant funds also offer significant advantages in terms of diversification and risk management:
Broad Market Exposure
Quant funds often employ strategies that allow for broad market exposure, investing across various asset classes, sectors, and geographies. This diversification helps to spread risk and reduce the impact of any single investment’s poor performance on the overall portfolio.
Advanced Risk Management Techniques
Quantitative models can incorporate advanced risk management techniques, such as stress testing and scenario analysis, to assess potential risks under different market conditions. These techniques enable quant funds to adjust their portfolios proactively, mitigating potential losses and enhancing overall stability.
Adaptive Strategies
Quant funds can adapt their strategies based on changing market conditions. By continuously analyzing data and refining their models, these funds can adjust their investment approach to align with evolving market dynamics, helping to manage risk more effectively.
Portfolio Optimization
Quantitative methods allow for precise portfolio optimization, balancing risk and return to achieve the desired investment objectives. By using techniques such as mean-variance optimization, quant funds can construct portfolios that maximize expected returns for a given level of risk, enhancing overall performance.
Challenges and Risks
Model Risk and Overfitting
Quantitative funds rely heavily on mathematical models to make investment decisions. These models are designed to identify patterns and predict future market movements based on historical data. However, one of the primary challenges associated with these models is the risk of overfitting. Overfitting occurs when a model is too complex and captures noise rather than the underlying signal in the data. This can lead to models that perform exceptionally well on historical data but fail to generalize to new, unseen data.
Overfitting can be particularly problematic in quant funds because it can result in significant financial losses. When a model is overfitted, it may suggest trades that appear profitable based on past data but are not effective in real-world scenarios. This risk is exacerbated by the fact that financial markets are dynamic and constantly evolving, meaning that patterns identified in historical data may not persist in the future.
To mitigate model risk and overfitting, quant funds often employ techniques such as cross-validation, regularization, and the use of out-of-sample testing. These methods help ensure that models are robust and capable of adapting to changing market conditions. Moreover, quant funds may also incorporate qualitative insights and expert judgment to complement their quantitative models, providing a more holistic approach to investment decision-making.
Market Volatility and Liquidity Concerns
Market volatility and liquidity are significant challenges for quant funds. Volatility refers to the degree of variation in the price of a financial instrument over time, and it can have a profound impact on the performance of quantitative models. High volatility can lead to rapid and unpredictable price movements, which may cause models to generate erroneous signals or fail to execute trades as intended.
Liquidity concerns arise when there is insufficient market activity to execute trades at desired prices. In illiquid markets, large trades can lead to significant price slippage, where the execution price differs from the expected price. This can erode the profitability of trades and increase transaction costs, ultimately affecting the fund’s overall performance.
Quant funds must carefully manage these risks by implementing robust risk management strategies. This may include setting strict limits on position sizes, diversifying across multiple asset classes and markets, and employing advanced algorithms to optimize trade execution. Additionally, quant funds often monitor market conditions closely and adjust their models and strategies in response to changes in volatility and liquidity, ensuring that they remain resilient in the face of market fluctuations.
The Role of Technology in Quant Funds
Machine Learning and AI
Machine learning and artificial intelligence (AI) have become integral components of quantitative funds, revolutionizing the way these funds operate and make investment decisions. Machine learning algorithms are designed to identify patterns and relationships within large datasets that may not be immediately apparent to human analysts. These algorithms can process vast amounts of data at high speeds, allowing quant funds to analyze market trends, predict asset price movements, and optimize portfolios with unprecedented accuracy.
AI technologies enable quant funds to adapt to changing market conditions by continuously learning from new data inputs. This adaptability is crucial in the fast-paced world of finance, where market dynamics can shift rapidly. AI-driven models can adjust their strategies in real-time, providing quant funds with a competitive edge over traditional investment approaches.
Moreover, machine learning techniques such as natural language processing (NLP) allow quant funds to analyze unstructured data sources, such as news articles, social media posts, and earnings call transcripts. By extracting sentiment and insights from these sources, quant funds can enhance their decision-making processes and gain a deeper understanding of market sentiment and potential risks.
Big Data and Computational Power
The rise of big data has transformed the landscape of quantitative investing, providing quant funds with access to an unprecedented volume and variety of information. Big data encompasses a wide range of data types, including structured data from financial markets, as well as unstructured data from alternative sources like satellite imagery, geolocation data, and social media activity. This wealth of information allows quant funds to develop more comprehensive and nuanced models for predicting market behavior.
To effectively harness big data, quant funds rely on advanced computational power. High-performance computing systems and cloud-based infrastructures enable the processing and analysis of massive datasets in real-time. This computational capability is essential for running complex simulations, backtesting strategies, and optimizing portfolios. The ability to process data quickly and efficiently allows quant funds to respond to market opportunities and risks with agility.
Furthermore, the integration of big data and computational power facilitates the development of sophisticated risk management frameworks. By analyzing diverse data sources, quant funds can identify potential risk factors and correlations that may not be evident through traditional analysis. This enhanced risk assessment capability helps quant funds to mitigate potential losses and improve overall portfolio performance.
Key Players in the Quant Fund Industry
Notable Firms and Influential Figures
Renaissance Technologies
Renaissance Technologies, founded by Jim Simons, is often considered the gold standard in quantitative investing. Known for its Medallion Fund, Renaissance has consistently delivered exceptional returns by leveraging complex mathematical models and algorithms. Jim Simons, a former mathematician and codebreaker, is a pivotal figure in the quant world, having pioneered many of the techniques used in quantitative trading today.
Two Sigma
Two Sigma, co-founded by David Siegel and John Overdeck, is another major player in the quant fund industry. The firm employs a diverse team of data scientists, mathematicians, and engineers to develop sophisticated trading strategies. Two Sigma is renowned for its use of machine learning and artificial intelligence to analyze vast datasets, allowing it to identify market inefficiencies and capitalize on them.
AQR Capital Management
AQR Capital Management, co-founded by Cliff Asness, is a prominent quant fund known for its research-driven approach. AQR integrates academic research with practical investment strategies, focusing on factors such as value, momentum, and quality. Cliff Asness is a well-respected figure in the industry, known for his contributions to the understanding of factor investing and risk management.
D.E. Shaw Group
Founded by David E. Shaw, the D.E. Shaw Group is a pioneer in the use of computational finance. The firm employs a multidisciplinary team to develop quantitative models that drive its investment strategies. David E. Shaw, a former computer science professor, is recognized for his innovative approach to trading and his early adoption of technology in finance.
Case Studies of Successful Quant Funds
Medallion Fund by Renaissance Technologies
The Medallion Fund is perhaps the most successful quant fund in history, boasting annualized returns of over 30% before fees. The fund’s success is attributed to its proprietary algorithms, which are constantly refined and updated. Renaissance Technologies’ ability to attract top talent and maintain a culture of secrecy has been crucial to the Medallion Fund’s sustained performance.
Two Sigma’s Risk Premia Strategy
Two Sigma’s Risk Premia Strategy is a notable example of a successful quant fund that leverages alternative data and machine learning. By identifying and exploiting risk premia across various asset classes, the fund has achieved impressive returns. Two Sigma’s emphasis on innovation and technology has allowed it to stay ahead of the competition in the rapidly evolving quant landscape.
AQR’s Style Premia Fund
AQR’s Style Premia Fund is a successful quant fund that focuses on capturing returns from well-documented investment styles, such as value, momentum, and carry. The fund’s systematic approach to investing, combined with rigorous risk management, has resulted in consistent performance. AQR’s commitment to research and transparency has made it a leader in the quant fund industry.
D.E. Shaw’s Composite Fund
The D.E. Shaw Composite Fund is a testament to the firm’s expertise in quantitative trading. By employing a diverse range of strategies, including statistical arbitrage and macro trading, the fund has delivered strong returns over the years. The D.E. Shaw Group’s focus on innovation and its ability to adapt to changing market conditions have been key to the Composite Fund’s success.
The Future of Quantitative Investing
Emerging Trends and Innovations
Quantitative investing is continuously evolving, driven by advancements in technology and data science. One of the most significant trends is the integration of artificial intelligence and machine learning into investment strategies. These technologies enable quant funds to process vast amounts of data more efficiently, uncovering patterns and insights that were previously inaccessible. Machine learning algorithms can adapt to new data, improving their predictive accuracy over time and allowing for more dynamic investment strategies.
Another emerging trend is the use of alternative data sources. Traditional financial data is being supplemented with non-traditional data, such as social media sentiment, satellite imagery, and transaction data, to gain a more comprehensive view of market conditions. This approach allows quant funds to identify investment opportunities and risks that may not be apparent through conventional data analysis.
The rise of quantum computing also holds potential for the future of quantitative investing. Quantum computers can process complex calculations at unprecedented speeds, potentially revolutionizing the way quant funds analyze data and execute trades. While still in its early stages, quantum computing could lead to significant breakthroughs in portfolio optimization and risk management.
Regulatory and Ethical Considerations
As quantitative investing becomes more sophisticated, it faces increasing scrutiny from regulators. The use of complex algorithms and alternative data raises questions about transparency and accountability. Regulators are concerned about the potential for market manipulation and systemic risk, prompting discussions about the need for more stringent oversight and disclosure requirements for quant funds.
Ethical considerations are also at the forefront of the future of quantitative investing. The use of alternative data, particularly data derived from social media and personal information, raises privacy concerns. Quant funds must navigate the ethical implications of using such data, ensuring that their practices comply with data protection regulations and respect individual privacy rights.
Moreover, the reliance on algorithms and machine learning models introduces the risk of bias and discrimination. Quant funds must be vigilant in monitoring their models for unintended biases that could lead to unfair or unethical investment decisions. This requires a commitment to ethical AI practices and ongoing evaluation of model performance and fairness.
In summary, the future of quantitative investing is shaped by technological advancements and the need to address regulatory and ethical challenges. As the industry continues to innovate, it must balance the pursuit of alpha with the responsibility to operate transparently and ethically.
Related posts:
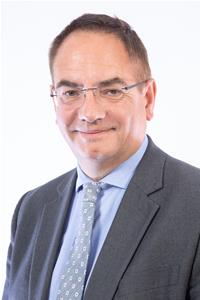
Adrian Lawrence FCA with over 25 years of experience as a finance leader and a Chartered Accountant, BSc graduate from Queen Mary College, University of London.
I help my clients achieve their growth and success goals by delivering value and results in areas such as Financial Modelling, Finance Raising, M&A, Due Diligence, cash flow management, and reporting. I am passionate about supporting SMEs and entrepreneurs with reliable and professional Chief Financial Officer or Finance Director services.